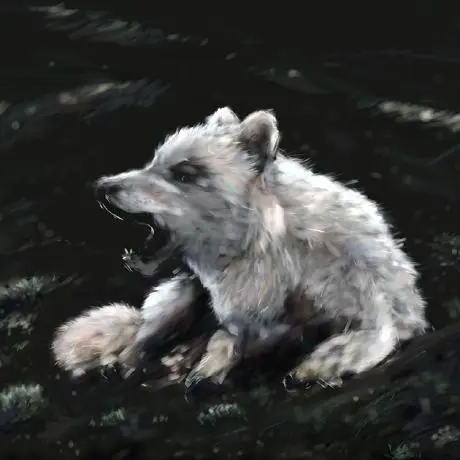

Ah, I see. I was going to recommend you a link to the audiobooks that I found.
I managed to find what I assume are English fansubs.
It was on Amazon Prime’s streaming service for a while so there should be official subs at least floating around.
https://github.com/KerfuffleV2 — various random open source projects.
Ah, I see. I was going to recommend you a link to the audiobooks that I found.
I managed to find what I assume are English fansubs.
It was on Amazon Prime’s streaming service for a while so there should be official subs at least floating around.
It’s fairly entertaining but you really have to suspend disbelief. I’d call it fantasy with some sci-fi jargon more than actual sci-fi. I guess I could say overall plot doesn’t make a lot of sense but scenery on the way isn’t too hard on the eyes.
Just curious, to you speak Mandarin?
Kind of reminds me of this skit about a guy kidnapped by a mermaid: https://piped.video/v/B-lRdR0AlSw
One would hope that IBM’s selling a product that has a higher success rate than a coinflip
Again, my point really doesn’t have anything to do with specific percentages. The point is that if some percentage of it is broken you aren’t going to know exactly which parts. Sure, some problems might be obvious but some might be very rare edge cases.
If 99% of my program works, the remaining 1% might be enough to not only make the program useless but actively harmful.
Evaluating which parts are broken is also not easy. I mean, if there was already someone who understood the whole system intimately and was an expert then you wouldn’t really need to rely on AI to port it.
Anyway, I’m not saying it’s impossible, or necessary not going to be worth it. Just that it is not an easy thing to make successful as an overall benefit. Also, issues like “some 1 in 100,000 edge case didn’t get handle successfully” are very hard to quantify since you don’t really know about those problems in advance, they aren’t apparent, the effects can be subtle and occur much later.
Kind of like burning petroleum. Free energy, sounds great! Just as long as you don’t count all side effects of extracting, refining and burning it.
So you might feed it your COBOL code and find it only coverts 40%.
I’m afraid you’re completely missing my point.
The system gives you a recommendation: that has a 50% chance of being correct.
Let’s say the system recommends converting 40% of the code base.
The system converts 40% of the code base. 50% of the converted result is correct.
50% is a random number picked out of thin air. The point is that what you end up with has a good chance of being incorrect and all the problems I mentioned originally apply.
I was speaking generally. In other words, the LLM will convert 100% of what you tell it to but only part of the result will be correct. That’s the problem.
Even if it only converts half of the codebase, that’s still a huge improvement.
The problem is it’ll convert 100% of the code base but (you hope) 50% of it will actually be correct. Which 50%? That’s left as an exercise to the reader. There’s no human, no plan, no logic necessarily to how it was converted also so it can be very difficult to understand code like that and you can’t ask the person who wrote why stuff is a certain way.
Understanding large, complex codebases one didn’t write is a difficult task even under pretty ideal conditions.
This sounds no different than the static analysis tools we’ve had for COBOL for some time now.
One difference is people might kind of understand how the static analysis tools we’ve had for some time now actually work. LLMs are basically a black box. You also can’t easily debug/fix a specific problem. The LLM produces wrong code in one particular case, what do you do? You can try performing fine tuning training with examples of the problem and what it should be but there’s no guarantee that won’t just change other stuff subtly and add a new issue for you to discovered at a future time.
Seems like we’re on the same page. The only thing I disagreed with before is saying the output was random.
It has to match the prompt and make as much sense as possible
So it’s specifically designed to make as much sense as possible.
and they should not be treated as ‘fact generating machines’.
You can’t really “generate” facts, only recognize them. :) I know what you mean though and I generally agree. I’m really interested in LLM stuff but I definitely don’t really trust them (and no one should currently anyway).
Why did this bot say that Hitler was a great leader? Because it was confused by some text that was fed into the model.
Most people are (rightfully) very hesitant to say anything positive about Hitler but he did accomplish some fairly impressive stuff. As horrible as their means were, Nazi Germany also advanced since quite a bit also. I am not saying it was justified, justifiable or good, but by a not entirely unreasonable definition of “great” he could qualify.
So I’d say it’s not really that it got confused, it’s that LLMs don’t understand being cautious about statements like that. I’d also say I prefer the LLM to “look” at stuff objectively and try to answer rather than responding to anything remotely questionable with “Sorry, Dave I can’t let you do that. There might be a sharp edge hidden somewhere and you could hurt yourself!” I hate being protected from myself without the ability to opt out.
I think part of the issue here is because the output from LLMs looks like a human might have wrote it people tend to anthropomorphize the LLM. They ask it for its best recipe using the ingredients bleach, water and kumquat jam and then are shocked when it gives them a recipe for bleach kumquat sauce.
It’s not supposed to be some enlightened, respectful, perfectly fair entity.
I’m with you so far.
It’s a tool for producing mostly random, grammatically correct text.
What? That’s certainly not the purpose of LLMs and a lot of work has been done to improve the accuracy of their answers.
Is it still not good enough to rely on? Maybe, but that doesn’t mean it’s just for producing random text.
What’s wrong with sea lions?
The graph actually looks like it’s saying the opposite. Fro most of the categories where there’s actually a decent span of time, it climbs rapidly and then slows down/levels off considerably. It makes sense also: when new technology is discovered, a breakthrough is made, a field opens up there’s going to be quite a bit of low-hanging fruit. So you get the initial step that wasn’t possible before and people scramble to participate. After a while though, incremental improvements get harder and harder to find and implement.
I’m not expecting progress with AI to stop, I’m not even saying it won’t be “rapid” but I do think we’re going to progress for the LLM stuff slow down compared to the last year or so unless something crazy like the Singularity happens.
It is only a matter of time before we’re running 40B+ parameters at home (casually).
I guess that’s kind of my problem. :) With 64GB RAM you can run 40, 65, 70B parameter quantized models pretty casually. It’s not super fast, but I don’t really have a specific “use case” so something like 600ms/token is acceptable. That being the case, how do I get excited about a 7B or 13B? It would have to be doing something really special that even bigger models can’t.
I assume they’ll be working on a Vicuna-70B 1.5 based on LLaMA to so I’ll definitely try that one out when it’s released assuming it performs well.
Is anyone using these small models for anything? I feel like an LLM snob but I don’t feel motivation to even look at anything less than 70-40B when it’s possible to use those models.
That seems like they left debugging code enabled/accessible.
No, this is actually a completely different type of problem. LLMs also aren’t code, and they aren’t manually configured/set up/written by humans. In fact, we kind of don’t really know what’s going on internally when performing inference with an LLM.
The actual software side of it is more like a video player that “plays” the LLM.
By “attack” they mean “jailbreak”. It’s also nothing like a buffer overflow.
The article is interesting though and the approach to generating these jailbreak prompts is creative. It looks a bit similar to the unspeakable tokens thing: https://www.vice.com/en/article/epzyva/ai-chatgpt-tokens-words-break-reddit
Are you using a distro with fairly recent packages? If not, then possibly you could try looking for supplementary sources that could provide more recent version. Just as an example, someone else mentioned having a similar issue on Debian. Debian tends to be very conservative about updating their packages and they may be quite outdated. (It’s possible to be on the other side of the problem, with fast moving distros like Arch but they also tend to fix stuff pretty fast as well.)
Possibly worth considering that hardware can also cause random crashes, faulty RAM, overheating GPUs, CPUs, memory or overclocking stuff beyond its limits. Try checking sensors to make sure temperatures are in a reasonable range, etc.
You can also try to determine if the times it crashes have anything in common or anything unusual is happening. I.E. playing graphics intensive games, hardware video decoding, that kind of thing. Some distros have out of memory process killers set up that have been known to be too aggressive, and processes like the WM that can control a lot of memory will sometimes be a juicy target for them.
As you probably already know if you’ve been using Linux for a while, diagnosing problems is usually a process of elimination. So you need to eliminate as many other possibilities as you can. Also, it’s general hard for people to help you with such limited information. We don’t know the specific CPU, GPU, distribution, versions of software, what you were doing when it occurred, anything like that. So we can’t eliminate many possibilities to give you more specific help. More information is almost always better when asking for technical help on the internet.
LibreWolf is a privacy oriented fork of FireFox. If you like FireFox, you could give it a shot.
That certainly sucks all the joy out of pirating it.